Guided diffusion has become the new go-to method for image generation. To avoid misuse of this inspiring new technology, we must ensure fake detection networks remain up to speed with recent developments. Using the approach described in “Diffusion models beat gans on image synthesis”.
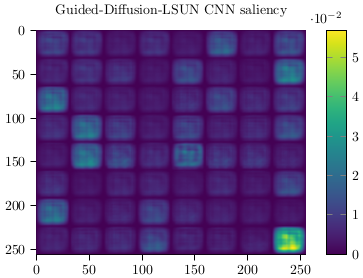
Wavelet packets decompose an input into blocks according to frequency. The blocks are arranged such that the frequency increases along the diagonal. The saliency plot reveals that the classifier relies on high-frequency information to spot the fakes.
Similarly, the plot below shows a classifier trained to identify GAN-generated. Once more the classifier runs on top of a wavelet packet representation. The classifier can identify the source with an accuracy of 95.85 ± 0.59%. The saliency map is shown below:
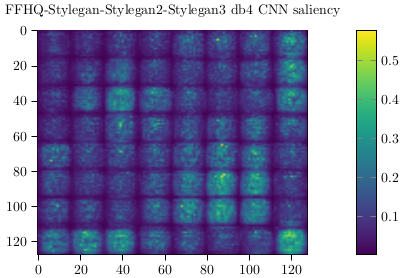
Again high-frequency information plays an important role when the classifier makes its call. This finding suggests that the methodology developed for GAN detection could also be useful for diffusor detection.
I am looking forward to seeing more research in this direction.
For more information on this topic and the method see our recent paper at rdcu.be/cUIRt .